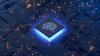
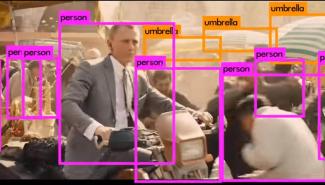
Yolo V5 - Object Detection
YoloV5 model released by Ultralytics for object detection. The component takes one image as input and outputs the coordinates of the bounding boxes of all objects detected in the image.
Physical AI refers to using AI techniques to solve problems that involve direct interaction with the physical world, e.g., by observing the world through sensors or by modifying the world through actuators. The data is generated from various sources, including physical sensors and ”human sources,” such as social networks or smartphones. Actuation may range from support to human decisions to managing automated devices(e.g., traffic lights, gates) and actively directing autonomous cars, drones, etc.
One intrinsic feature of Physical AI is the uncertainty associated with the acquired information, its incompleteness, and the uncertainty about the effects of actions over (physical) systems that share the environment with humans. In other words, Physical AI deals with unreliable, heterogeneous, and high-dimensional sources of data/information and a significant set of actuation variables/actions to learn models, detect events, or classify situations, to name just a few cases. In some cases, a decision-making loop is closed over physical systems with their dynamics, often complicated and challenging to model (e.g., weather dynamics, human crowd behavior).
To tackle such large physical problems, existing techniques for data processing and decision-making are not tractable. Thus, one should develop and improve methods that exploit redundancy, combine/infer partial/missing data, transfer knowledge (e.g., through learning) and exploit low-rank characteristics of data to reduce the several relevant dimensions of the problems (in terms of observation, state and action spaces).
YoloV5 model released by Ultralytics for object detection. The component takes one image as input and outputs the coordinates of the bounding boxes of all objects detected in the image.
Backend of EO4NOWCAST Near Real-Time Soil Moisture Assessment and Pluvial Flood Nowcasting services
A neural network-based time-series forecasting model for concentrations of an electrochemical reaction.
Tag-My-Outfit is a classifier that predicts the category and the attributes of a piece of clothing viewed in a given image. It is containerized and gRPC enabled so that it can be used standalone or in a pipeline using AI4EU experiments platform.
A data set of 1.8 billion measurements from a mechanical wrist with three axes that can hold tools, for example, for spray painting in combination with a pump. The data set spans six months in 1-second intervals.
An AI resource to track "group-leader" social interactions within videos
Reinforcement Learning (RL) and State Representation Learning (SRL) Toolbox for Robotics
Speech recognition translates spoken information into digital text in real time
A decision-making framework for active perception with POMDPs.
This dataset contains hyperspectral images of denim fabric over 224 reflectance bands. It was used to test automated composition analysis in the DIH4AI Open Call 2 FABCOD project (Fabric Composition Detector).
This file contains a synthetic dataset to create baseline behavior models for transformers temperature. The dataset is a 5 columns containing the 4 temperature and the hour, with a 5 minutes frequency.