A simple guide to Physical AI
This page is meant to be an accessible entry point to what is meant by “Physical AI”, and to the resources on Physical AI that are available in the AI4EU AI on-demand platform. This guide is part of the broader AI4EU scientific vision on “Human-centered AI”, available here.
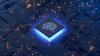
What is “Physical AI”
Physical AI refers to the use of AI techniques to solve problems that involve direct interaction with the physical world, e.g., by observing the world through sensors or by modifying the world through actuators.
Physical AI thus aims at solving real-world problems that require the ability to observe and collect data in (possibly very large) environments; model and integrate such heterogeneous data into representations suitable for automated reasoning, for example by robots, to decide actions; or simply for supporting humans in their daily decisions.
One intrinsic feature of Physical AI is the uncertainty associated with the acquired information, its incompleteness and the uncertainty about the effects of actions over (physical) systems that share the environment with humans. What distinguishes Physical AI systems is their direct interaction with the physical world, contrasting with other AI types, e.g., financial recommendation systems (where AI is between the human and a database); chatbots (where AI interacts with the human via Internet); or AI chess-players (where a human moves the chess pieces and reports the chess board state to the AI algorithm).
Taking the robotics realm as an example, the range of applications and “intelligence” of currently available robots is very large. On one hand of the spectrum, traditional industrial robots perform repetitive operations in automated shop-floors, requiring little interaction with the environment and/or humans through sensing, such as welding, assembling or machining. On the other end, service robots interacting with humans rely significantly on their sensors (e.g., to navigate using a map or landmarks, to find objects and manipulate them, to recognize humans and interact with them), and the results of their actions are not always as expected, given the complexity of the environment they are dealing with. Such robots are examples of systems that not only require intelligence to handle an unpredictable world but also use that intelligence to process data from physical sensors, and act physically in the world.
But Physical AI systems are not limited to robotics. One can consider a physical AI system extending pollution sensing capabilities in cities by networks of less expensive mobile micro-sensors, installed, for example, in municipal electrical cars. Their information can be used to estimate and/or classify pollution levels directly from sensor data and/or feed mathematical models. The cars transporting the sensors can even be actively directed to paths that provide extra information, using suitable algorithms for decision-making. AI techniques can help make pollution models more precise, augmenting them with new ways of sensing and understanding. Mobile polluting sources like cars and target crowds can be counted from city cameras and widespread pollutant sources like home ovens can be ”mined” from images collected in shopping or real estate websites. Besides clustering city areas by their level of pollutants/health risk, such a physical data-driven system would provide support for decision-making in at least two major ways: a) suggesting directions to people to avoid risky zones (e.g., through apps used by asthmatic people, or by attracting people to non-polluted areas through event advertisement) and b) operating gates/traffic signs to open/close routes to designated areas, so as to manage the pollution level distribution.
Another example concerns mobile robot systems wirelessly networked with sensors and actuators, e.g., in hospital or home scenarios. The robots need to process information from multiple onboard (e.g., microphones, touch pads, cameras, laser scanners) and offboard networked sensors (e.g., cameras, photocells, motion detectors, microphone arrays) to build awareness of the state of the system, and use their own (e.g., manipulator arms, speakers, expressive LCD screens) or networked (light switches, motorized blinds, automated door locks) actuators to perform the required tasks while ensuring proper navigation and interaction with humans. In the hospital domain, tasks can consist of transporting meals and medicine from/to rooms of the hospital, or playing interactive games with children in pediatric wards or particular pilot sessions. At home, a multi-purpose robot can dialogue with the home owners using speech, so as to perform tasks such as picking up objects from other rooms, remotely switching lights, blinds or other devices(e.g., fridges, TV sets), and also hosting and handling differently visitors such as the postman, the food delivery person or the medical doctor.
Please help us to complete and maintain this document by notifying corrections or addition to the document maintainers, João Paulo Costeira and Pedro Lima.
Note: if you want to add a software resource, data set or researcher to this document, you first need to make sure that they are available in the AI4EU platform, e.g., by publishing the software.
This document is published under the Creative Commons License Attribution 4.0 International (CC BY 4.0). It should be cited as:
João Paulo Costeira and Pedro Lima (editors), “A simple guide to Physical AI”. Published on the AI4EU platform: http://ai4eu.eu. June 24, 2020.