POMDP-IR Solver
A decision-making framework for active perception with POMDPs.
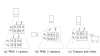
Main Characteristic
Partially Observable Markov Decision Processes with Information Rewards (POMDP-IR) is a framework to compute policies for autonomous agents with the goal of gathering information about particular features on the environment. SymbolicPerseus-IR extends one of the most knowns POMDP solvers to include Information Rewards. It lets you compute and test policies for a given input environment model.
Research areas
Physical AI
Technical Categories
Planning and scheduling
Last updated
09.03.2022 - 17:23
Trustworthy AI
The SymbolicPerseus-IR library computes the best policies for autonomous agents given an input environment model. The safety and trustworthiness of AI agents should be guaranteed by the user designing the model.
GDPR Requirements
The SymbolicPerseus-IR library does not collect or use any data from users.