SUMO-RL
Traffic Control in a Simulated Environment for Pollution Reduction
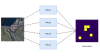
Main Characteristic
SUMO/RL implements a pipeline with a traffic simulator of the city of Trondheim, Norway, and a reinforcement learning autonomous agent that learns and implements traffic control policies with the goal of minimizing the number of pollution peaks above a given threshold. Each component can be ran stand alone.
Research areas
Physical AI
Technical Categories
Machine learning
Business Categories
Transportation
Last updated
15.06.2021 - 19:45
Detailed Description
Additional information: This asset implements two docker containers, developed to interact with each other, although each can be ran stand alone, i.e., the simulator can be used to collect data about pollution patterns and accepts any other kind of agent, while the agent can be used with simulators of different cities.
External links:
- Github repository: https://github.com/Mika412/AI4EU-RL-Trondheim
Trustworthy AI
SUMO/RL computes and implements policies for traffic control in a simulated environment. Safety and trustworthy of AI agents should be guaranteed by the user if and when transfering the policies to a real environment.
GDPR Requirements
SUMO/RL does not collect or use any data from users.