PLANET (hyPer Local climAte driven Tool)
Climate change, with its accompanying global warming and increasingly severe weather conditions, presents substantial risks to agricultural sustainability worldwide. This is driving the agri-food sector to adapt through the use of new technologies and structural changes to production. Adaptation strategies include everything from the introduction of weather-resistant crops and new livestock breeds to altering crop rotation patterns and building protective structures for livestock. Despite these efforts, the complexities of climate change mean the impacts vary across regions and over time. Policymakers are also working to support the sector, but challenges such as a lack of observational data on agricultural climate shocks, insufficient stakeholder participation in policy-making, and slow policy performance evaluations contribute to suboptimal policy formulation. Given this context, the immediate development of methodologies and tools that facilitate better climate adaptation decision-making for all agri-stakeholders is vital. Addressing this need, the PLANET project proposes to harness technologies such as Artificial Intelligence, Big Data Analytics, and the wealth of geospatial earth observation data from the EU Copernicus and Eumetsat Agencies. The objective is to create an intelligent, automated tool that provides on-demand, hyperlocal climate-driven land-use suitability services.
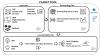
Α Climate Risk assessments with a Decision Support Tool for Land suitability : A process for evaluating the potential impacts of climate change on a particular region, sector, or activity. The PLANET services include A Generative Adversarial Network that downscales the climate information and a feedforward neural network that classifies the land suitability for specific crops based on the refined input data. The linked asset presents the results of the PLANET tool, executed for the three use cases: Kenya, Papua New Guinea, Puerto Rico.
The asset provides input/output of the PLANET tool for each of the executed steps. Firstly, the AOI is defined in txt format, which triggers the docker image of STEP1 of the PLANET tool that downloads the necessary land and climate data specific to the AOI. Secondly, the downloaded data is stored, and the climate module in STEP2 downscales the climate information. Thirdly, STEP3 executes the Long Short-Term Memory (LSTM) that uses Normalized Difference Vegetation Index (NDVI), precipitation and temperature data, and yield from the FAOSTAT (Food and Agriculture Organization STATistics) database to make an estimation of the next year's yield. The NDVI is calculated using the AI4COPERNICUS service. Finally, STEP4 executes the classification of land suitability based on thresholds for climate variables and soil health. The climate input is from STEP2, downscaled at higher resolutions, and soil health data is obtained from the SoilGrids database.
To request a demo of the tool please contact Neuralio A.I.