LORE
LORE (LOcal Rule-based Explanations) is a model-agnostic explanator for tabular data
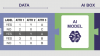
Daniele Fadda, CNR
Main Characteristic
LORE is a model-agnostic explanator capable of producing rules to provide insight on the motivation a AI-based black box provides a specific outcome for an input instance.
Research areas
Explainable AI
Business Categories
Earth Observation
Last updated
04.06.2021 - 12:54
Detailed Description
The method of LORE does not make any assumption on the classifier that is used for labeling. The approach used by LORE exploits the exploration of a neighborhood of the input instance, based on a genetic algorithm to generate synthetic instances, to learn a local transparent model, which can be interpreted locally by the analyst.
Documents
Trustworthy AI
The explanation layer implemented in LORE is intended to augment the trust and confidence of the analyst towards AI-based decision support systems. In particular, for black-box decision systems, the system may improve the insight of the analyst on the internal strategy of the AI algorithm.
GDPR Requirements
The method provides an explanator capable of creating an explanation in the form of logical rules. This approach addresses the "explicability" requirement of the GDPR, where a requirement is fixed for automated decision processes that have an impact on humans.