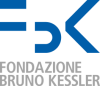
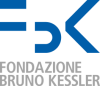
We use binary supervised learning for discovering process models as temporal logic patterns
As the need to understand and formalise business processes into a model has grown over the last years, the process discovery research field has gained more and more importance, developing besides approaches for discovering procedural models also approaches for discovering declarative models. Orthogonally to this classification, the vast majority of works envisage the discovery task as a one-class supervised learning process guided by the traces that are recorded into an input log.
In this work instead, we focus on declarative processes described through temporal logic formulae and embrace the view of process discovery as a binary supervised learning task, where the input log reports both examples of the normal system execution, and traces representing “strange” behaviours according to the domain semantics. We therefore deepen how the valuable information brought by both these two sets can be extracted and formalised into a model that is “optimal” according to user-defined goals.
The proposed approach, namely NegDis, is also evaluated with respect to other relevant works in this field, and shows promising results as regards both the performance and the quality of the obtained solution.
The microproject involves FBK and the University of Bologna, as well as the Free University of Bolzano as external partner.
Tool for the discovery of temporal-logic patterns as a binary supervised learning problem
Synthetic event logs generated starting from a Declare process model related to a Loan Application scenario composed of positive and negative traces