Stereotypes in Language & Computational Language Models
Stereotypes in language refer to the biases and generalizations embedded in linguistic expressions, which can perpetuate societal prejudices based on factors like gender, race, ethnicity, or social status. Computational language models, such as OpenAI's GPT-3.5, have come under scrutiny for their potential to absorb and reinforce these stereotypes. This raises concerns about the marginalization of certain groups, the amplification of biases, and the perpetuation of systemic injustices. Efforts are being made to mitigate biases in language models through techniques like bias detection, debiasing algorithms, and inclusive data collection. Collaboration among researchers, developers, and diverse communities is vital in creating language models that are more aware, fair, and respectful, fostering inclusivity and progress in our society.
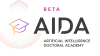
In these two interdisciplinary projects, Katrin Schulz and Robert van Rooij have combined knowledge from linguistics, psychology, and natural language processing. In one project they study whether and how generic sentences — which typically express (perhaps stereotypical) generalisations — should be interpreted, and whether corresponding implicit generalisations can be found in computational language models trained by (huge) linguistic corpora. The main hypothesis is that the generalisations that we accept are due to how such generalisations are learned and that similar ideas are behind the learned language models. In the other project, the main object of study is the impact of (online) media on stereotypical beliefs people hold. In order to do so, it will be measured which stereotypes are expressed by computational language models and how such biases relate to stereotypical beliefs that individuals may hold. Another goal is to develop a computational model to understand how media sources affect stereotypical beliefs.