Recurrent Neural Networks. LSTMs Lecture
Nowadays, Artificial Intelligence drives scientific and economic growth worldwide. This is largely due to advances in Machine Learning (ML), notably in Deep Neural Networks (DNNs), which are essentially massive ‘learning by experience/examples’ systems. Their applications span and revolutionize almost every human activity:
-Autonomous Systems (cars, drones, vessels),
-Media Content and Art Creation (including fake data creation/detection), Social Media Analytics,
-Medical Imaging and Diagnosis,
-Financial Engineering (forecasting and analytics), Big Data Analytics,
-Broadcasting, Internet and Communications,
-Robotics/Control
-Intelligent Human-Machine Interaction, Anthropocentric (human-centered)Computing,
-Smart Cities/Buildings and Assisted living.
-Scientific Modeling and Analytics.
Several DNN advances and challenges hit the news almost every day, arising discussions on AI ethics, privacy protection and its societal impact.
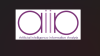
This lecture overviews Recurrent Neural Networks and Long Short-Term Memory (LSTM) networks that have many applications in signal and video analysis. It covers the following topics in detail: Neural Networks for Sequence Analysis. Recurrent Neural Networks and their relation to State space filters. RNN forward propagation. Activation functions. Loss functions and RNN training through Back-Propagation. Long Short-Term Memory. LSTM forward propagation. Gated Recurrent Unit. GRU forward propagation. It also overviews the following applications: Image Captioning, Object tracking, and Image denoising.