Probability Theory Lecture
Many CVML scientists, engineers and enthusiasts do not have solid mathematical background, as it is so easy to jump into almost any CVML domain using available libraries and frameworks. This is very much true in Deep Learning and leads to a cacophony of inaccurate statements and a polyphony of ill-defined terms and concept. Therefore, a rigorous mathematical background is a must for anybody working in this area. Luckily, most ECE/CS curricula provide such foundations.
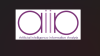
This lecture overviews Probability Theory that has many applications in a multitude of scientific and engineering disciplines, notably in Pattern Recognition and Machine Learning. It covers the following topics in detail:
- a) Probability Space, Bayes theorem.
- b) One random variable, cumulative probability functions, probability density functions, expectation operators, mean, variance, functions of random variables, normal, uniform, Laplacian distributions.
- b) Two random variables, joint cumulative probability functions, joint probability density functions, expectation operators, independence, correlation coefficient, functions of two random variables, 2D normal, uniform, Laplacian distributions.
- c) Multiple Random Variables, random vectors, joint cumulative probability functions, joint probability density functions, expectation operators, independence, correlation matrix, covariance matrix, functions of two random variables, multivariate normal distributions.
Finally, a section is devoted on random number and random vector generation.