Geometry Lecture
Many CVML scientists, engineers and enthusiasts do not have solid mathematical background, as it is so easy to jump into almost any CVML domain using available libraries and frameworks. This is very much true in Deep Learning and leads to a cacophony of inaccurate statements and a polyphony of ill-defined terms and concept. Therefore, a rigorous mathematical background is a must for anybody working in this area. Luckily, most ECE/CS curricula provide such foundations.
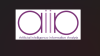
This lecture overviews Geometry that has many applications in Computer Vision and Machine Learning. It covers the following topics in detail: Vector calculus (inner/cross vector products, coplanarity), 3D geometric transformations (rotation, translation,quarternions), Projective geometry: homogenous coordinates, Perspective (or central) projections, Vanishing points, Cross-ratio, Conic sections.