Domain Adaptation Lecture
Nowadays, Artificial Intelligence, notably Advanced Machine Learning (ML) drives scientific and economic growth worldwide. They are essentially massive ‘learning by experience/examples’ systems. However, as our tasks and the world change, such systems should adapt to new domains/tasks and continue learning. Knowledge should be transferred from one DNN systems to other ones. Distributed DNN training should be performed though Federated Learning, e.g., for privacy protection. New Learning modes should be explored, by reward maximation, as it is done in Deep Reinforcement Learning and Imitation Learning.
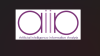
This lecture overviews Domain Adaptation that has many applications in DNN training and adaptation. It covers the following topics in detail: Domain Shift, Unsupervised Domain Adaptation (Domain-specific Whitening Transform, Min-entropy Consensus loss, Maximum Classification Discrepancy, Sliced Wasserstein Discrepancy), Deep Learning methods for Unsupervised Domain Adaptation ( DLID: Deep learning for DA by Interpolating between Domains), Semi-supervised Adaptation (Entropy minimization, minmax entropy), Supervised Domain Adaptation, Bayesian Domain Adaptation, Margin Disparity Discrepancy.