AI4Agriculture
Precision agriculture aims at analysing (near) real-time data to predict, improve and optimise the production and the quality of farming and to reduce the environmental impact of agriculture. The application of AI techniques can help farmers to improve their products and their benefits while reducing the environmental footprint.
The AI4Agriculture pilot is targeting a specific application to help predicting the yield and assessing the quality of the production in vineyards using remote sensing data and AI, therefore showcasing the AI4EU platform in the context of agriculture.
Categories
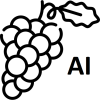
AI4Agriculture in a nutshell
In a nutshell, the AI4Agriculture pilot addresses the following objectives:
- Vineyards need to know in advance (predictions) the estimated yield production and its potential quality.
- Help on the decision-making process based on data (i.e. prune the vines to respect the production quota or to decide the parcels assigned to produce wines of different quality).
- Need of a knowledge base to provide mapping services to be integrated into third-party apps
The pilot value proposition of the pilot is aiming at:
- Delivering AI models using satellite, drone and mobile data (images) to predict the production and quality.
- Provide data integrated into a Knowledge Graph and annotations for model training.
- Predict to plan ahead and take actions (i.e. planning right harvesting time or intervention in the parcels), which may lead to costs savings and quality improvements.
- Deliver the solution to an early adopter and offer data and models and showcasing the European AI on-demand platform.
Pilot walkthrough
The architecture provides an overview of the different elements of the pilot:.
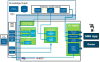
First of all, a few selected land parcels were reserved by the vineyards collaborating with the experiment. These are the places where the mobile and drone pictures were taken, each area represented by a different level of production: high, medium and low, based on the production results from previous years. The pilot developed a mobile app to be able to take the images from the field, annotate them with observations (e.g. the number of clusters of grapes observed in the field for the plant in the picture), and upload the images to a server for further processing.
Based on the data collected in the summer of 2020, a counting model to detect the number of clusters of grapes in the mobile pictures was developed and trained. Another model to calculate the multiespectral index for plant vigour (NDVI) was developed in parallel to determine the amount of foliage that occludes the clusters of grapes, based on the pictures taken by the drones and satellite data.
Other variables (i.e. soil, plant variety, laboratory and wine analyses, weather conditions history, etc.) have been also collected. All these data have been consolidated into a knowledge base in RDF along with an ontology that models the results and a SPARQL endpoint to access to the data.
With all this info, a machine learning model has been trained to predict the yield and several quality indicators (i.e. pH, total acidity, etc.). These data has been crossed with real production from the vineyard to check the predictions.
Finally, a web app to visualize the results of the models over an interactive map. The results of these can be seen in the figure below:
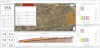
Exploitation of the AI4Europe platform
The pilot started in the second year of the project and run since before the summer 2020 to the harvesting after summer 2021, up to November 2021. Due to the asynchronous nature of the different models and the methodology, the pilot decided to make use of the AI4EU Experiments mainly to deliver the models, but without creating specific AI pipelines.
The pilot is nevertheless delivering several results to the platform, including models, data and other resources. All resources have been tagged with the “AI4Agri” tag to enable an easy search of the artifacts related to the pilot. Below there is a list of elements available in the platform:
AI4EU Experiments:
- Fruit counting model:
- Yield and Quality model
- NDVI model
- Graphical User Interface to launch models
AI Catalog (resources):
- Mobile images dataset
- Drone images dataset
- Annotations of mobile images
- RDF dataset (knowledge graph) and ontology
Cooperation between the organizations
In order to deliver a coherent pilot, the interaction among the pilot partners has been crucial.
- ATOS led the pilot, setting up conference calls and meetings and contributions to WP6. From the technical perspective, ATOS developed the yield and quality models and led the integration and execution of the models into a coherent proof-of-concept application, including a graphical user interface to serve as hub of all the components of the pilot.
- Smart Rural (SMR), as an SME developing intelligent solutions for farmers, led the requirements, data collection from drones and mobile, and validation of the overall solution of the pilot. SMR engaged with three vineyards at the Ribera del Duero wine region (Martín Berdugo, Pradorey and Vizcarra) to ensure the data gathering and testing. SMR is also interested in the exploitation of the results.
- DLR has a proven track record on analysing satellite data, especially Copernicus (Sentinel) images, and drone data. Therefore, DLR led the access to remote sensing algorithms and provided the NDVI model for plant vigour.
- Based on their previous expertise in computer vision in different domains, including agriculture, the Universitat Politècnica de Catalunya (UPC) developed the fruit counting model to determine the areas of the mobile images covered by grapes’ clusters and led the methodology for labelling the mobile images to train the model.
- The University of Athens (UoA) provided expertise on data modelling, data storage, data fusion and data interlinking using tools based in the usage of linked data and knowledge graphs, as well as visualizations using their tools (e.g. Sextant).
Further Work
The pilot has been conceived as an end-to-end proof of concept of what can be achieved in a smart farming setting using AI and delivered through the AI4EU platform. The goal was not to create a fully-fledged application ready to be used in the real world, but rather to pave the way to future research and showcasing the platform in the scope of precision agriculture.
The models delivered can be improved with further observations and re-trained to improve their performance. We knew that only two years of data is not enough to have very performant models, so we created a methodology for labelling that might serve to improve some of the models (e.g. the counting model) in the future. In the case of the quality and yield prediction, the models are calculated, re-trained and executed any time new data arrives, in a reinforcement learning fashion.
Our partner SMR is interested on exploiting this type of solution in their applications, as they are dealing with customers in the smart farming ecosystem.