WildCapture
Dataset for Out-of-distribution detection
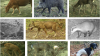
The dataset used in this study represents a valuable resource for the task of animal species classification in a real-world scenario. Comprising more than 60k images from 15 distinct classes, each representing different animal species.
These species include: Beech Marten, Crested Porcupine, Domestic Cattle, Domestic Dog, Domestic Horse, Eurasian Badger, European Hare, European Roe Deer, Grey Wolf, Persian Fallow Deer, Red Deer, Red Fox, Western Polecat, Wild Boar, and Wild Cat.
The dataset used in this study represents a valuable resource for the task of animal species classification in a real-world scenario. Comprising more than 60k images from 15 distinct classes, each representing different animal species. These species include: Beech Marten, Crested Porcupine, Domestic Cattle, Domestic Dog, Domestic Horse, Eurasian Badger, European Hare, European Roe Deer, Grey Wolf, Persian Fallow Deer, Red Deer, Red Fox, Western Polecat, Wild Boar, and Wild Cat. The dataset was collected using CameraTrap technology, providing authentic and diverse wildlife imagery. To ensure accurate annotations, each image underwent handcrafted annotation by domain experts, guaranteeing high-quality ground truth labels. Moreover, it encompasses a diverse range of lighting conditions, including both daylight and nocturnal images. We present a selection of examples from our dataset, highlighting various species and lighting conditions. This broad spectrum of lighting scenarios allows for a more thorough evaluation of model generalization and adaptability in real-world scenarios.
To enhance the dataset's utility for both classification and out-of-distribution (OOD) detection, we employed the MegaDetector framework to annotate bounding boxes (bbox) around each animal depicted in the images. This step facilitated the cropping of individual animal instances during the training process, leading to improved model performance in recognizing fine-grained details. This dataset allowed us to explore the effectiveness of our OOD detection approach, as we randomly split the dataset to create distinct OOD classes not encountered during the training phase.
By leveraging this dataset, we provide a robust evaluation platform for our novel approach. The dataset's comprehensive representation of various animal species, combined with expert annotations and bbox information, ensures the reliability and realism of our experimental results, highlighting the potential of our OOD detection technique in real-world wildlife applications.
If you use this dataset please cite: Cultrera, Luca, Lorenzo Seidenari, and Alberto Del Bimbo. "Leveraging Visual Attention for out-of-distribution Detection." Proceedings of the IEEE/CVF International Conference on Computer Vision Workshops. 2023.