AI for network loads and demand forecasting towards efficient operational planning
A prediction engine that optimizes operational planning and reserve allocation costs and overall improving reliability of the grid operations.
Categories
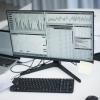
What is the challenge that is being addressed?
The massive penetration of distributed renewable energy resources and an expected more proactive behaviour of consumers by taking part in demand response initiatives are, among others, key drivers that significantly increase the complexity in which the power systems will be operated and planned. System operators need to develop methodologies to assess both current and expected system behaviours. In that regard, load prediction is of paramount importance for the overall assessment of the network.
What is the AI solution the project plans to implement?
This pilot aims to exploit the availability of R&D NESTER’s historical load consumption data, using an ensemble for short-term load forecasting that implements online forecasting and relies on the combination of physical and ML approaches into a hybrid approach. It uses statistical signal processing techniques to assimilate heterogeneous data. This pilot aims to leverage the adaptation of different types of ML methods, namely Support Vector Machines, Random Forests and Deep Learning, using a self-adaptive forecasting ensemble. The system performs online forecasting, i.e. the most recent measurements compliant with the forecast horizon are used as features to forecast. It enables continuous error evaluation, which is an insurance against non-stationarity Associated with document Ref. Ares(2020)7592177 - 14/12/2020 101016508 I-NERGY – PART B 22 of the data generating processes. The engine will rely on external data (e.g. weather) to improve the load forecasts by using clustering techniques to create specific models to different climate/seasonal patterns.
This pilot will provide a prediction engine that rely on models built from the extensive historical data available. The engine will continuously collect the most recent data in order to output periodical load forecasts with time-horizons of 2h and 36h ahead and with 15mins time steps. Main expected benefits consist in improving the accuracy of load forecasts for the intra-day and day-ahead horizons of the operational planning tools. This prediction engine aims to be used permanently as decision support tool for the control room dispatcher, allowing thus to complement, in an automatic way, the information available in a period compatible with operational planning procedures. Consequentially it will optimize operational planning and reserve allocation costs and overall improving reliability of the grid operations.
Who will help implement this solution?
This pilot is implemented within the framework of the “I-NΕRGΥ: Artificial Intelligence for Next Generation Energy” Project. The I-NERGY Project has received funding from the European Union's Horizon 2020 Research and Innovation programme under grant agreement No. 101016508.
The responsible partner for this Use Case is R&D Nester (https://www.rdnester.com/en-GB).
Learn more about I-NERGY pilots here: https://i-nergy.eu/pilots
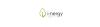